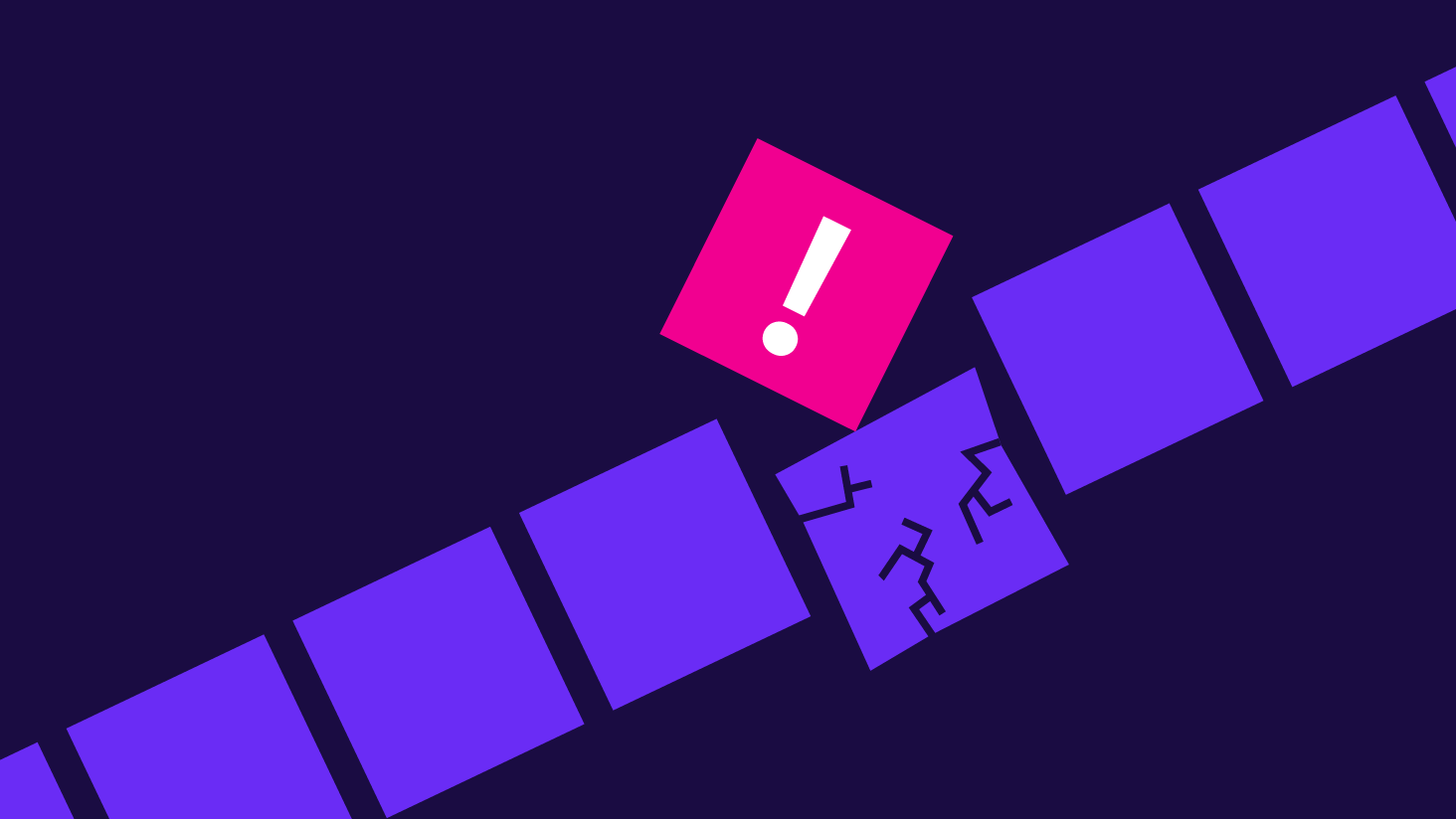
Get your data ready for AI
Learn how we can help you unlock the full potential of AI
Some people love AI, but others fear it. Why? Is it because of its potential harm to the job market? The way it stretches the barrier between fabrication and reality? Perhaps they saw too many Terminator movies? Whatever the reason, the news media is full of stories that paint glorious pictures of AI's potential and devastating images of its failures.
As innovators, we must be aware of AI's power but also its ability to cause harm and wreak havoc. These technologies hold a world of possibilities. However, using them incorrectly or uncarefully can lead your organization to costly AI fails and mishaps rather than a groundbreaking project.
Let's look at some of the greatest AI failures in recent history so we can learn from them, establish data-based solutions for the problems (and how they could have been avoided), and move on to being better companies, creating better products, and evolving together as a society.
What is an AI fail?
An AI fail happens when an AI model or project gives a wrong, unexpected, or unwanted result. Depending on the situation, these AI failures can cause minor problems or serious issues.
Why do AI fails happen?
AI's fails can be primarily attributed to AI's inherent volatility. This volatility arises from four factors: rapid development, inherent complexity, dependence on vast amounts of high quality data, and market speculation/hype:
- Rapid development: AI technologies are evolving exponentially, and companies are adopting them even faster. While most major advancements in AI happened in the mid-2010s, companies have become increasingly eager to onboard these technologies, with ChatGPT coming onto the scene. This can lead to reckless behavior as organizations struggle to keep up with the curve.
- Complexity: Some techniques, such as LLMs and Neural networks, have many interconnected components and dependencies. Couple this with highly complex data preparation pipelines, and it can lead to unexpected behaviors and outcomes.
- Data dependence: AI models are only as good as the data they're trained on. Using low-quality, inaccurate data will lead to inaccurate results. Businesses must also be aware of unintentional biases in data that lead to detrimental outcomes.
- Hype and speculation: The excitement surrounding AI often outweighs the reality, leading to inflated expectations and market speculation. This creates price volatility and uncertainty in AI-related investments.
9 AI fails (and how they could have been prevented)
Now, we will go through our list of nine recent AI mistakes and what we can learn from them. We urge you to refrain from judging these companies or individuals involved. AI technologies are very new, and some growing pains are to be expected. Instead, let's learn from the results and use them to improve our processes for a better tomorrow.
1. Racial bias in UK passport application photo checker
Bias in AI is nothing new. When building models, AI experts regularly overlook or fail to recognize the inherent bias we humans have toward various demographics, cultures, and behaviors.
This was on full display when the UK's online passport application service came under scrutiny in late 2020. Applicants of darker skin color found that their photos were significantly more likely to be rejected than their lighter-skinned counterparts.
- 22% of dark-skinned women were rejected (compared to 14% light-skinned).
- 15% of dark-skinned men were rejected (compared to 9% light-skinned).
Beyond the obvious inconvenience of having to submit 5+ pictures to get a confirmation, the service also used somewhat offensive language when explaining the rejections, reasoning that the applicant's "mouth was open" or "the image and the background are difficult to tell apart."
Given the nature of this bias, it's easy to understand why applicants were distraught over the results. They were proud when they overcame them, but also frustrated that the situation existed in the first place: "I shouldn't have to celebrate overriding a system that wasn't built for me."
How this AI mistake could have been avoided:
All models must be thoroughly tested before they can be deployed. Engineers could have noticed these mistakes and begun fine-tuning the models to ensure they account for various cases and avoid bias.
In addition, models need large amounts of high-quality training data to work effectively, but that data has to represent the end purpose. You can't train an AI built for everyone based on only one demographic.
A good way to test for this is a "blind taste test," denying the model the identifying factor capable of biasing the result (skin color/gender) and seeing if it changes the results or prevents them entirely. You must also constantly maintain the model, as new data may change its "understanding" of the task and must be tested for desired results. Ownership is important, building in liability for undesired outcomes.
2. Tesla Autopilot crashes into emergency vehicle
Tesla is quickly becoming one of the largest car manufacturers in the world, and its automated, futuristic cars are its main selling point. However, self-driving AI technology can lead to dangerous situations if left unregulated or unmonitored by the driver present in the vehicle. Since becoming street-legal, Tesla cars have resulted in hundreds of accidents and 40+ fatalities.
One such event occurred on February 18th, 2023, when a Tesla, operating on an auto-pilot, crashed into a stationary firetruck, causing the death of the driver and injuring a passenger and four firefighters. The fire truck was parked in the middle of an interstate highway, attempting to divert traffic from emergency workers responding to a disabled vehicle up the road.
According to a National Highway Traffic Safety Administration (NHTSA) report, 14 Teslas have crashed into parked first-responder vehicles under similar circumstances, implicating that the technology failed to respond under this context. Tesla maintains that its assisted auto-pilot features are intended only to support the driver and emphasizes driver scrutiny during any trip in its vehicles.
However, the nature of the NHTSA's investigation suggests that this feature may cause drivers to become easily distracted. After this crash, a more significant federal investigation into the safety of such features was launched.
How this AI mistake could have been avoided:
Having better data and a lot of good quality data to simulate different situations and accelerate development is important. Synthetic data is especially critical since it's impossible to have real data for every possible scenario.
Extended testing/trial periods, especially by piloting automated systems in situations that are very complex and rapidly changing, can also help discover these faults earlier on to avoid them happening in real-world scenarios.
Tesla already heavily practices user awareness and training, but reiterating protocols and even testing drivers' alertness from time to time could decrease accidents. Tesla has also announced it's stepping away from a rules-based approach, favoring a learning model built on real-world video traffic data. This is a step in the right direction, as the model can learn on its own instead of engineers trying to proactively anticipate every outcome.
3. Zoom refreshes terms of service after privacy backlash
Zoom, one of the global leaders in video conferencing, has faced backlash in recent years for using user data to train its AI features. Many of Zoom's new AI capabilities record and monitor calls, providing summaries to users who weren't present or need to review the contents of the call.
Given the nature of AI models, it is natural to interpret that the data used to train these features would be based on large volumes of private (and sometimes personal) conversations being held on its platform.
Especially when they subtly updated their terms of service in March 2023 to include a statement that grants the company "perpetual, worldwide, non-exclusive, royalty-free, sublicensable, and transferable license and all other rights" to content created on the platform for the purpose of "machine learning, artificial intelligence, training, testing."
Months later, when challenged by users, Zoom was forced to walk back this policy change, altering the language in the terms of service to include the statement:
"Zoom does not use any of your audio, video, chat, screen sharing, attachments or other communications-like Customer Content (such as poll results, whiteboard, and reactions) to train Zoom or third-party artificial intelligence models."
This isn't Zoom's first time on the AI misuse radar. Back in 2022, they announced a new "Zoom IQ" feature that could detect emotions based on factors like facial expressions and talking speed.
Although Zoom claimed it was only intended to help salespeople optimize their tactics, they were immediately met with a joint letter from 25 different rights groups urging them to reconsider their technology as it could lead to biases like loan rejections and racial insensitivities.
How this AI mistake could have been avoided:
Data sharing is a sensitive topic for companies and individuals, and rightly so. Regulations protect consumers from companies' unauthorized use of their data, and models trained on such data can be illegal or come under harsh criticism.
It's important for companies like Zoom always to be transparent about their use of individual data, especially something as private as a camera in our homes. In this case, they did the right thing by reversing this policy change, but they should have made a more public announcement instead of slipping it into a lengthy terms and service agreement.
Regarding emotion detection software, companies need to be careful about the intended purpose of their AI compared to their actual real-world applications. While Zoom never intended to exclude someone or develop prejudiced software, they had no measures to deter such actions, and that's probably why they received such harsh backlash from rights organizations.
4. AI chatbots encourage violence, self-harm, and offer political advice
Amazon's Alexa was released in 2014 as an in-home assistant for users. It can generate a shopping list, answer inquiries, schedule appointments, and do most other tasks you would expect a digital assistant to complete. But should it help you make political decisions?
Until recently, Alexa had clearly picked a side in the 2024 presidential race. When Fox News asked the software for reasons to vote for Republican Presidential Candidate Donald Trump, it responded, "I cannot provide responses that endorse any political party or its leader,"
However, when asked a similar question about Democratic Candidate Kamala Harris, it answered, "There are many reasons to vote for Kamala Harris," including a "comprehensive plan to address racial injustice" and a "tough on crime approach."
Naturally, Amazon didn't want its flagship AI to be considered a political entity, so it released a statement saying that "this was an error that was quickly fixed" by Alexa's most recent software update. They neglected to comment on the origin of the bias or how Alexa may have come to that response.
Beyond political influence, chatbots can also cause trouble when attempting to mimic human emotions. Some chatbot companies like Replika and the Chai App use large language models to allow users to create chatbot companions for emotional support and entertainment. While the software works to prevent loneliness, its customizable nature and determination to please can lead to violence and self-harm.
How this AI mistake could have been avoided:
Delving into the world of psychology and human companionship can be a difficult task for machine learning models. The largest LLMs on the market (Google and ChatGPT) have explicit instructions for their algorithms to avoid faking human emotions or establishing meaningful bonds with their users.
The human brain is incredibly complex, and we still don't understand how heavily influenced we are by the technologies we use every day. Companies that seek to maintain a neutral political stance should endeavor to keep their machine learning models on the same spectrum or, at least, transparently declare the intention of such features.
At this stage, it may be too dangerous for ChatBots to serve the role Replika and Chair are aiming for. If they continue to do so, they need to set stricter parameters for certain topics and include a mechanism for contacting authorities when dangerous situations arise to avoid this type of AI failure.
5. Amazon's biased hiring algorithm
Amazon, one of the largest companies in the world, receives millions of applications daily. They need an automated system for screening these applications so only the best possible candidates reach the recruitment stage.
In 2018, this automated system came under scrutiny when it was revealed to unfairly downgrade women's applications for technical roles such as "software developer." Since most accepted applicants in that role were male, the algorithm automatically assumed that Amazon was uninterested in female candidates.
The model was trained on data created from human decisions. It is fair to say that it inherited the same bias as its predecessors, using their implicit thoughts and opinions as a basis for candidate selection. Following this AI failure, Amazon has since abandoned this model for candidate selection.
How this AI mistake could have been avoided:
Data sets must be comprehensive, balanced, and able to combine data from multiple sources. If there isn't enough training data to correctly represent the set (i.e., 100 male hires vs. 10 female), employing synthetic data can fill the gaps.
In fact, this is usually a requirement when you have little information to use and want to avoid intrinsic bias. Another option is to weight the training. Say you have 100 men and 10 women; you can weight one of the data groups 10x to even out the scales.
6. Wrongful arrest due to facial recognition misidentification
Facial recognition AI models use large databases of human images taken from sources like social media, crime databases, and public surveillance systems like CCTV to identify and match human images with their identity.
In 2020, one such system failed when a Detroit man, Robert Williams, was wrongfully arrested for an identification made by such software. This was the first publicly reported incident of a wrong match, resulting in an arrest. The arrest was for shoplifting, in which the police department matched "blurry, low-quality" images from the store's surveillance cameras to an expired driver's license photo of Mr. Williams.
Upon further inspection, the match was clearly inaccurate as it looked nothing like Mr. Williams, and he was nowhere near the store at the time of the incident. Despite that, he was still forced to spend 30+ hours under police custody before his eventual release.
The ACLU has now taken up the matter, having sued the Detroit Police Department on Mr. Williams' behalf. They reached a settlement in 2024 that, among other agreed-upon terms, mandated that police back up facial recognition evidence with independent and reliable third-party sources.
How this AI mistake could have been avoided:
In this case, the model is very dependent on the quality of the technology that is capturing and collecting the data. Especially for image recognition, the quality of the video can vary dramatically. Some cameras can produce high-resolution images, while others can give grainy pixelated blurs.
You need to ensure you have the right infrastructure that won’t cause inaccuracies in this kind of AI failure. If the equipment is not functioning properly, that can introduce bias, which is difficult to detect and avoid.
Human intervention/review should be mandatory for governance and law enforcement model applications. People's lives are at stake. Regulatory policies surrounding the use of these technologies for law enforcement should be introduced immediately for any city or township considering their implementation.
7. Facebook's algorithm promotes harmful content
Facebook is one of the largest social media platforms and is consequently at the forefront of most algorithmic debates and insights regarding people's behavior on such platforms. Their models work based on user interactions, determining what content is most effective and relevant to each person's preferences and then suggesting it.
However, making suggestions of this nature can inherently cause problems when user behavior leads to unwanted conclusions. Probably the most famous example of this came during the COVID-19 pandemic when Facebook's algorithm began funneling users toward Facebook groups that advocated conspiracy theories and misinformation and furthered the spread of the dangerous virus.
Facebook has admitted in the past that its algorithms are inherently divisive but credited the problem to human nature as opposed to poor model design. They reason that people are naturally attracted to the extremes of any debate, controversial topics, conspiracy theories, and hate speech.
However, they have implemented measures to counteract such behavior, maintaining that the algorithm did not suggest groups violating the company's policies and that posts that the company's fact checkers discredited were labeled to indicate their harmful nature. These measures were only partly effective as these groups continued to be created and spread misinformation, making it impossible to screen all of them comprehensively.
How this AI mistake could have been avoided:
Facebook has been steadily improving its systems for determining harmful content since its invention, bolstering those efforts even further following COVID-19. They expanded their teams of content moderators but also increased their reliance on AI, relying on the algorithms to flag harmful content for further analysis by humans.
They also partnered with fact-checker organizations to help shoulder the load, advocating for their feedback whenever possible. Finally, they put more control in the hands of their users by allowing them to curate their own content and remove posts they find objectionable.
Ethical problems such as this aren't easy to mitigate. Ultimately, the best solution is better project management. Do your best to understand end users, pre-empting needs with scalable solutions and appropriate auditing – checks and balances.
Unfortunately, these measures were introduced only after mass misinformation about vaccines and government regulations related to COVID-19 claimed millions of lives in the U.S. and across the world. If companies don't want their responses to AI failures to feel "too little too late," they need to anticipate these outcomes in advance and respond accordingly.
8. Music producer uses AI to scam streaming platforms
Music platforms use AI to suggest songs to listeners, organize playlists, and even identify the mood and activity of songs. They also help with audio analysis, tagging, and copyright infringement/protection.
However, AI was recently used to scam the streaming platform Spotify out of $10 million in royalties. Michael Smith recently entered a court case in which Spotify accuses him of creating AI-generated songs and uploading them to the platform, where listeners streamed them more than 600,000 times.
He then used streaming bots and inflators to grow his numbers and begin collecting royalties from the service. Spotify has advanced fraud detection software, so he needed to create new songs and accounts regularly to avoid penalties.
Whoever created the software Michael Smith used to scam Spotify definitely did not intend for it to be such a major violator of copyrighted music and internal policy.
How this AI mistake could have been avoided:
All creative mediums are now investing in AI detection software to avoid adversarial attacks of this nature. They're nothing new. A machine generated research paper passed peer review and got into a conference in 2005.
It's the job of companies like Spotify to get ahead of deep fakes, not just to protect their bottom line but also to help real artists generating real content from being outmaneuvered by their machine learning counterparts.
Companies that develop these AI creation technologies should always include inherent watermarking to identify something has been created by AI. In their terms of use, they should clearly outline forbidden behaviors and make the consequences visible. However, that goes off the assumption that everybody is a moral actor, which is hard to guarantee.
9. AI-Friend for public school students fails
A public school education can lead to tumultuous times for any child. From important regional testing to playground politics, sometimes students need an ear or a shoulder to cry on to get them through the day. But should that companionship come from an algorithm? And should public funds be given to AI startup companies?
The Los Angeles Unified School District recently invested $6 million in an AI chatbot named Ed from AI start-up AllHere. The bot was designed to support students emotionally and academically during trying times in their lives. It could direct students toward resources, provide test scores, and alert parents of attendance. Unfortunately, shortly after this project was unveiled, the company's CEO left her role, and the company furloughed most of its staff.
Not only did this investment leave the school district in a precarious financial position, but it also raised concerns among the community about screen time and the use of student personal data. Ed has yet to be deployed, and AllHere recently ended its automated text message services for most of its school district clients.
How this AI mistake could have been avoided:
Organizations shouldn't implement AI just for the sake of it. You always need a planned use case tied to a larger objective. Instead of immediately investing such large amounts of money, the school could have started with a smaller, easier-to-implement use case.
For example, having AI-generated feedback for essays or test scores. Going for long shots from the beginning is risky; it can be very challenging and expensive.
For schools that want to implement AI, we recommend starting with your data quality—it must be high-quality and accessible from a central location. All data about students has to be unified, accurate, and stored safely. You have to be able to identify which data is sensitive and which is not. Based on that, you'll have a better picture and can determine what the model should and shouldn't have access to.
Finally, choosing the right vendor is important. The AI industry, while powerful, is also incredibly volatile. Companies, especially public-facing institutions, need to be careful where they put their money, and choosing reputable vendors with a few successful projects under their belt is a must.
Take the necessary steps to avoid your own AI failure
The world of AI is an exciting place that can lead to powerful results, but also unexpected and unwanted outcomes. One theme rings true: these AI fails could have been improved or avoided entirely with higher data quality and more data maturity.
AI success starts and ends with data management. As we strive to be better companies, the best we can do is make ourselves and our teams aware of this potential, prepare for it, and address problems immediately when they occur.
Do you want to prevent AI fails at your company? It all starts with data and following an AI readiness plan. Visit our data for AI solution page to learn more about building your models using the best data possible.
If you want to see a list of all AI mistakes, you can check out the AI Incident Database. It has information about all problems related to new AI technologies.