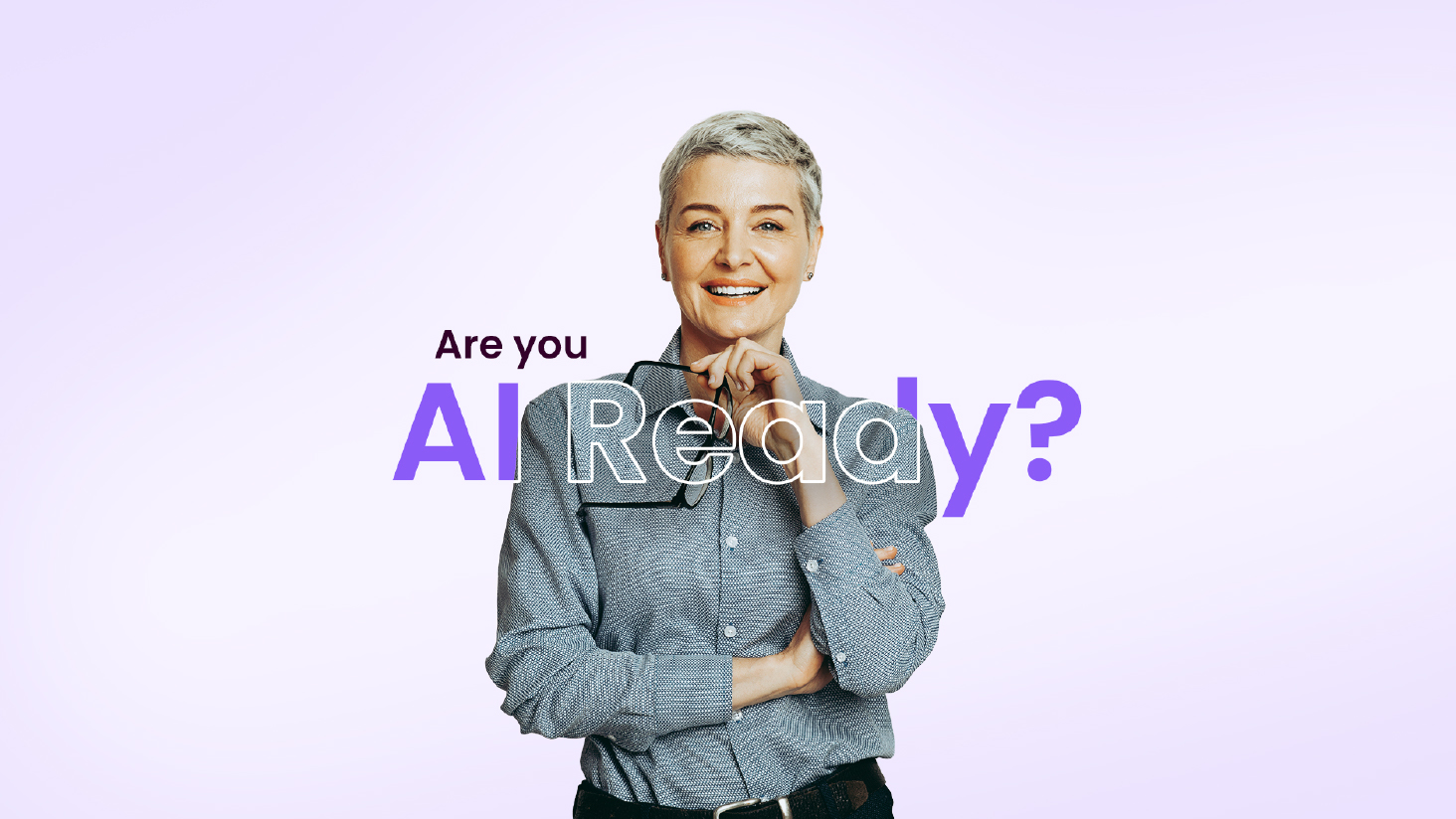
See the
platform
in action
Recently, Ataccama hosted a LinkedIn live on the topic of AI readiness where I got the opportunity to speak with Dima Turchyn, AI Product Marketing Lead at Microsoft, CEMA, and exchanges learnings from working with customers at different stages of their AI journey. We explored the hype and opportunity of this once-in-a-decade disruptive technology to answer the question: Are you AI-ready?
Continue reading to get my top takeaways from the discussion!
I'm a huge Formula One fan, and I find every element of motorsport thrilling. So, when we talk about AI and what companies need to do to implement it successfully, I immediately think of the analogy of the pace car and the race car.
The pace car is the data strategy. It might not be the most exciting part of the race, but it ensures the track is in the right condition and safe; without it, the race can't start. The AI strategy is the race car, which is the star attraction, the main event that everyone is interested in, but it cannot start the race until the pace car has done its job.
Quality data is the essential foundation for successful AI initiatives, and getting this combination right will distinguish the winners from the runners-up in today's competitive business landscape.
It's no surprise, therefore, that the organizations that have made an active effort to prioritize data governance, quality, and accessibility over the last few years are set to be the AI groundbreakers of today. But what about everybody else — how do they catch up?
#1 Who's leading the AI charge, and what can we learn from them?
Let's look at some examples of companies leading the way in delivering AI initiatives.
- Lean innovation companies: These organizations prioritize technology as a core component of their vision and leadership. They actively seek out AI solutions to transform their products and services, embracing new technologies as they emerge, and typically have a simplified data landscape.
- Regulated industries (e.g., Financial Services): Companies in sectors with stringent regulations, such as financial services, are often early AI adopters. They leverage AI to enhance customer service, streamline operations, and improve decision-making while adhering to compliance requirements.
- Government and Public Sector: Government agencies, often rich in publicly available data, are increasingly turning to AI to transform citizen services. AI-powered solutions help improve efficiency, personalize interactions, and optimize resource allocation.
Whether for regulatory, operational, or ambitious reasons, their data projects have left them better equipped to leverage AI's full potential and become the early adopters leading the charge. This is why they're performing so well in the nascent AI landscape.
To keep pace with these leaders, companies must invest in their data infrastructure and foster a culture that embraces data democratization, preparing their systems and culture for their heavily data-reliant AI future.
#2 The stakes are a lot higher when your data is used for AI
Businesses are pushing aggressively to adopt AI to automate and improve their most critical business processes, such as providing AI solutions for customer service and modernizing their supply chain. It makes sense to use the most powerful tools for the most important functions. However, with great power comes greater responsibility.
To become a data-driven organization, the Chief Data Officer needs to drive the culture shift and infuse AI proactively throughout the organization. The CDO role and the data strategy they define will be critical to the success of their AI strategy.
Driving this are the data scientists and data engineers who need access to data to train the AI models that will be used to automate these business processes. However, 80 percent of their work is still spent finding, accessing, understanding, and wrangling the enterprise's data and preparing it for use in their AI initiatives.
To do this, Dima emphasized the need to figure out what exact data the models should use — and what they should not. Businesses need to behave responsibly towards their customers' data while also ensuring it can deliver trustworthy insights.
Unstructured data adds further complexity, with models processing speech, vision, and tables. Ensuring quality is essential as poor data will deliver inaccurate analytics, and consequences could include taking the business or innovation in the wrong (and expensive) direction, disrupting the supply chain, or delivering poor customer service. I mentioned how "one wrong statement in an LLM-powered digital assistant to your customers can have serious consequences on your brand and your wallet."
Because the stakes are so high, Dima outlined a change he had noticed in what organizations are looking for when choosing AI vendors, which I found interesting. Instead of prioritizing speed, efficiency, and price, businesses focus on the model type, its ability to catch incorrect behavior (undesired outputs), and a guarantee that responses are grounded in the data it's been built on.
Therefore, organizations need to pay close attention to how they build and implement these processes, and it all begins with the data they use. It is vital that there is a focus on aligning your AI strategy with your data strategy and implementing proactive measures to improve and prepare your data before feeding it into the models.
This way, you can avoid these risks and build applications with peace of mind, knowing they were built on high-quality, trusted data and will deliver the desired results with minimal hiccups.
#3 Quality data should be your top priority
Dima and I outlined five things companies must keep in mind when getting AI ready:
- Technology and culture. When creating a data strategy, remember that cultural change is just as important as adopting the relevant technology. Companies can get lost in a million different data management solutions. Still, they won't have any success if you can't get your team members, decision-makers, and everyone else on board.
- Proactive data strategies. Many companies try to respond to data issues retroactively, fixing them as they occur. By implementing proactive data management tools (like data observability), you can prevent issues before they cause any real damage and better position yourself for big data projects (like AI adoption).
- Test and learn. Focus on getting high levels of trust in data so you can start experimenting with use cases, helping you identify and fix issues early on. The initial pain will pay off in the long term.
- Keep everything flexible and remain patient. Dima made an excellent point about these models' volatility and continued improvement. It's fair to say that in the next six to twelve months, we will see significant improvements and changes to these systems. That's why it's important to remain vigilant, continue to improve, but also have the flexibility to change when necessary.
- CDOs are evolving. Another point Dima and I agreed on was the ever-changing role of the CDO. Moving beyond data management, CDOs are increasingly seen as catalysts for AI initiatives within their organizations. They are responsible not only for ensuring data quality and governance but also for shaping AI strategies, promoting AI adoption across departments, and establishing ethical frameworks for AI use. As AI becomes more integral to business operations, CDOs must use their expertise and leadership to drive innovation and maximize the value of AI investments.
Ready to unlock the full potential of AI for your organization?
So, are you AI-ready? If you want to get the most out of your AI applications, Ataccama can help. Head here for more insights and advice.