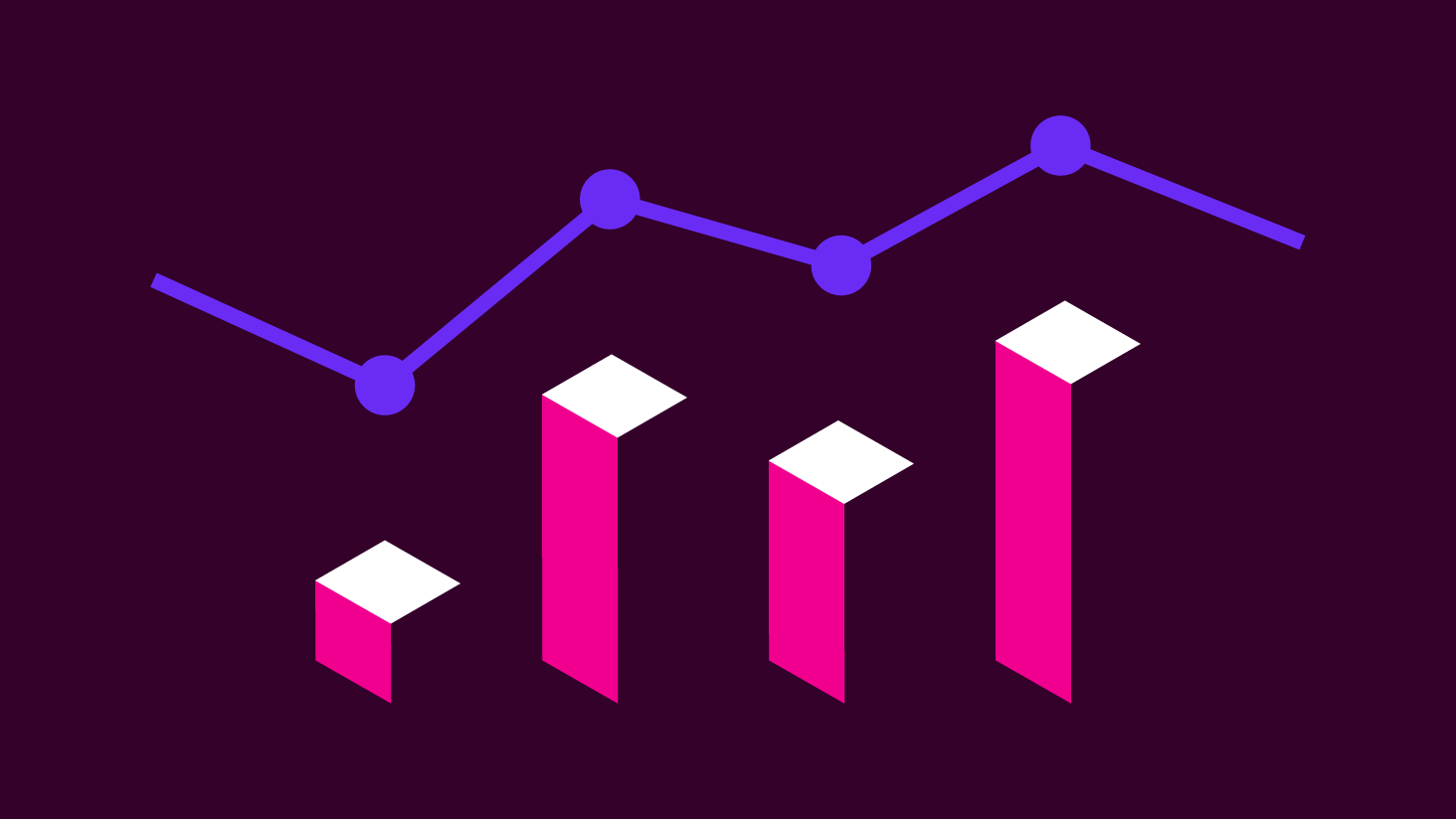
See the
platform
in action
Today, most businesses collect data. Whether it's shipping routes or customer profiles, companies need data quality metrics to help determine the value of this information. Depending on the results, you can discover if your data is underutilized or even unlock its hidden potential.
But what are the most critical data quality metrics? And what is the best way to measure and keep track of them? In this blog, we'll dive into nine important data quality metrics. Let's get started.
What are data quality metrics, and why do they matter?
Data quality metrics are the various measurements companies use to assess their ability to handle data quality and produce valuable and useful data. Depending on the company, the volume of data, and the systems where it's stored, these metrics can vary significantly but come down to seven key dimensions regarding the data:
- Accuracy. Is the data accurate?
- Completeness. Is the data complete or missing some values?
- Consistency. Is the data consistent with other records organized in the same way?
- Timeliness. Has the data been updated recently and delivered efficiently?
- Validity. Can the data be verified?
- Uniqueness. Is the data unique, or does it have duplicates?
- Relevance. Is the data representative of the information you're looking for?
Concerning the importance of data quality, tracking these dimensions is just as important as collecting the data itself. Without performing a regular data quality assessment to ensure all of these dimensions are ideal, you risk poor data reaching downstream systems, leading to costly errors and bad data quality throughout the organization.
Therefore, data quality metrics assess your organization's ability to preserve these dimensions and deliver records that fulfill expectations and meet data quality KPIs.
What are the most important data quality metrics to track?
While the characteristics above are useful, they are more symptoms than actual sources of the problem – overall descriptions as opposed to specific functions and tasks.
To achieve high data quality, you need to assess the actual elements of your data systems and use those to build reports on your ability to achieve these characteristics.
Below are nine data quality measures to help your organization achieve and improve your overall data quality score.
1. Data downtime (DDT)
Data downtime refers to times in the company when data is partial, hard to find, inaccurate, or entirely unusable. It is the one circumstance that data-driven organizations strive to avoid and is compiled of three smaller metrics: number of incidents, time-to-detection, and time-to-resolution.
- Number of incidents. How many data incidents happen over a set period.
- Detection time. How long it takes to detect a data incident.
- Resolution time. How long it takes to resolve an incident.
By evaluating these three factors, you will get a clear picture of your data quality management system and its ability to hit data quality KPIs. It doesn't have so much to do with the data itself as your ability to address and resolve issues when they inevitably occur.
2. Table uptime
Access to your data tables is essential for their benefit to your business. Table uptime measures how often your data tables are accessible and useful to stakeholders, measuring their accessibility and usability over a period of time.
It's calculated by dividing the total time a table was available and functioning property over a designated time frame. For example, if your table was available for 12 out of 24 hours, its table uptime would be 50%.
3. Importance score
To effectively prioritize data quality issues, you must know which tables/data points in your organization are the most important. You can do this by assigning each record an "importance score," which factors in the number of read/write actions on each entry and the overall downstream consumption of the table at the business intelligence level.
4. Table health
Table health measures the frequency and number of incidents a table experiences over time. Tables with a lower health score need more attention or should be avoided until their health improves.
5. Table coverage
This measures your systems coverage and ability to assess data quality across the entire system. A comprehensive platform like data observability will give you automated insight into all your tables with real time updates on the key dimensions.
6. Custom monitors on key tables
For your company's most important tables, you'll need to create custom monitors to measure unique dimensions and better monitor their critical functions. You'll also need to set up alerts so your system can notify the right people when problems arise.
The number of custom monitors you have on key tables can also provide insight for a data quality assessment.
7. Unused tables and dashboards
An organization with high data maturity will get the most out of its data systems. This means that if you have many unused tables and dashboards, it can indicate missed opportunities. "Days since last write" is a good way to determine if a table is being used regularly or not.
8. Deteriorating queries
If a query's execution run time increases over time, it's known as a "deteriorating query," meaning something is slowing it down or lowering its efficiency. Once you see a query deteriorating, addressing it immediately before it becomes a full-fledged data issue is essential.
9. Status update rate
The rate at which your status updates will directly affect your knowledge of your data system. If you're not updating regularly enough, you risk missing data issues, giving them a greater chance to cause actual harm to the company.
This mainly concerns your alert strategy and its effectiveness in getting data teams to respond to incidents immediately.
How to measure data quality: 8 steps
Now that we know some key metrics to monitor, let's examine how to assess data quality in your organization.
Step 1: Establish clear objectives
If you're still learning how to measure data quality, establishing clear goals and data quality KPIs for your organization is the best place to start. Set three-, six-, and twelve-month goals based on the metrics and dimensions mentioned above to define what you want to achieve with data quality tracking.
Step 2: Set benchmarks
Once your data quality measures are in place and you've defined your objectives, it's time to set benchmarks to ensure you're on the right track. This all begins with assessing the current state of your data quality and then setting realistic benchmarks based on your capabilities, the tools you have available, and your expectations for the program.
Step 3: Select your key metrics
While all the above metrics are valuable information, they aren't universal. Your company may have only a few tables to keep track of, so something like table coverage or unused tables might not be as relevant to you. Each use case is unique. By defining what's most important, you can prevent losing sight of your priorities.
Step 4: Choose data sources
It's difficult to track data quality measures if you don't know where your data comes from. One of the most critical steps in any data quality initiative is cataloging data sources so issues can be traced back to their origin.
Step 5: Use tools for assistance
Any data quality initiative would be handicapped without an adept data quality tool for streamlining these tasks. Most of the information mentioned above should be available in your data catalog, while a data quality tool, like Ataccama ONE, can measure data quality dimensions and deliver those reports directly inside the catalog.
Step 6: Data analysis
Once data has been onboarded onto your DQ tool, you must work with it to recognize patterns and other items stakeholders should keep in mind. Is it following your expectations? What's causing these issues? Can you use the collected information to improve or solve the problem?
Step 7: Reporting and visualization
Now that your data quality measures are in place, you'll need a way to deliver this information to relevant stakeholders. Using your report catalog is a good starting point, and providing the information in easy-to-digest charts (data visualization) will expand the number of users who can contribute to the process as a whole.
Step 8: Continuously monitor and refine processes
Data quality monitoring is an ongoing process that needs regular attention to be done correctly. Companies must routinely assess and adjust their data quality processes to refine them and adapt to changes to data systems as they inevitably occur.
Prove the value of DQ with data quality metrics!
Tracking data quality metrics is essential for organizational success because it ensures your data is valuable and usable. Regularly performing a data quality assessment and monitoring metrics like accuracy, completeness, consistency, and timeliness can identify and address data issues before they lead to costly errors and bad data across your organization.
This proactive approach helps maintain high-quality data, crucial for making informed decisions, improving operational efficiency, and achieving business goals.
A comprehensive data quality platform, like Ataccama ONE, can automate this process and provide real-time insights into your data's health.
Look at our What is data quality and why is it important? Ultimate guide that dives into everything you need to know about the world of data quality.