How to Design UX for AI and Chat Assistants
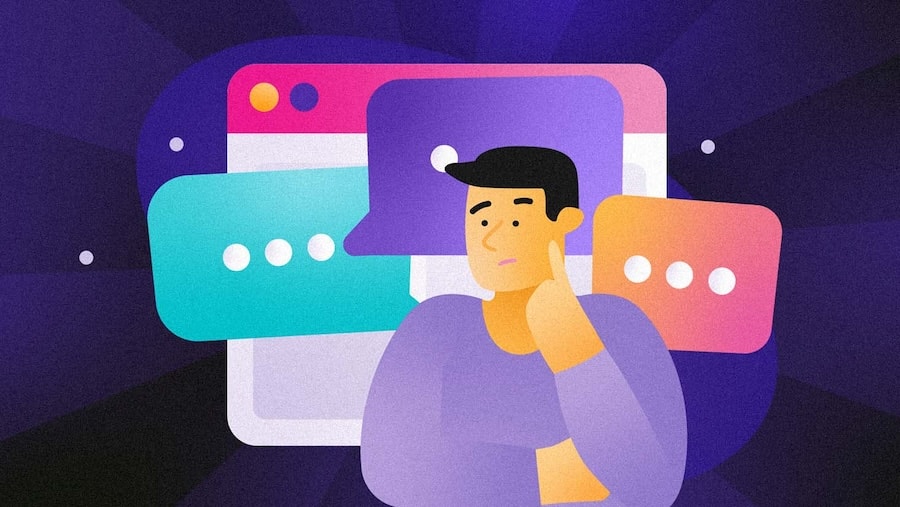
ChatGPT is a new and exciting technology that has taken the world by storm in recent months. While it holds seemingly limitless potential in terms of real-world applications, the design principles of its interface have been in place for many years. They can hold the key to making this tool more effective and universally accepted.
Many of these principles link back to the fundamentals of AI and how people perceive it. By carefully adjusting AI characteristics like explainability and anthropomorphism (and using traditional UX methods as references), we can create better applications with broad benefits for our companies and industries.
Because I’m a UX researcher, my first step is always to start with the user. Read on to learn the advantages and disadvantages of popular UX designs for AI and valuable advice on implementing the best UX for your AI and chat assistants.
UX of conversational interfaces
Let’s discuss the nature of these interfaces. Was Chat GPT the first iteration of these models? Are chat interfaces effective for all use cases? Should you anthropomorphize your assistant? I’ll answer all these questions and then offer advice for designing user interfaces for these systems.
From full-text search to question-answer systems to chatbots
Concerning interaction style, ChatGPT is merely an evolution of existing systems that have been actively used (e.g., research organizations, customer support, corporate bots) in recent years with a big boom of chatbots around 2016 — they have a text field input and an enter key, but is this enough?
In contrast to ChatGPT, question-answer systems such as IBM Watson Assistant were created with a specific use case, a user problem, and a need in mind.
Thanks to this, they have well-defined inputs and outputs (e.g., input suggestions and explanation of answers) but are limited to individual products. They are, therefore, more usable for users to solve specific user/business problems. All these systems are carefully tuned for their purpose, guaranteeing correct/defined and explained answers.
Compared to them, ChatGPT is a powerful “toy,” but we still don’t know what it’s good for.
Are chat interfaces a good idea?
Designing for chat or voice interfaces has its specifics due to the de facto absence of a user interface – interaction, therefore, starts to approach the command line, but without strict rules on command syntax (e.g., recognition vs. recall). Operations such as back, redo, edit, return to the beginning, and local or global help must be carefully designed by the conversational designer. This ensures that the system’s responses provide truthful and meaningful information in the given context and that conversation navigation is comfortable for users.
In some situations, no display is available; all interaction relies on the user’s short-term memory. The designer must also create and describe a natural language recognition model for each user’s intent (and here, large language models “LLMs” can help the most), but the answer must be correct and truthful in the given context (e.g., terms of services of an e-shop differ from each other and a generated LLM response cannot be used).
This raises the question of which use cases chat interfaces are suitable for and where their benefits outweigh the drawbacks. Today, these are predominantly scenarios where the input is not implicitly defined, such as customer support (FAQs/complaints), chatbots for psychological assistance, navigation (although here, the interaction from the user is significantly limited with input through a touch display), or smart homes. These are scenarios where chatbots or conversational agents benefit from creating a relationship with the user.
Should your agent be anthropomorphized?
Designers often want their chat assistants to have a personality. They favor models that have been “anthropomorphized,” and look and sound like actual human conversation. The personality of the conversational agent, tone, and voice can draw users into the interaction, build a relationship, and ultimately lead to long-term use and increased user satisfaction. Moreover, the literature also shows that adding anthropomorphism to the conversational agent can increase user trust in the entire system.
However, designing an agent or system in this way has some disadvantages. If an anthropomorphized agent fails (provides incorrect information), it can create a sense of betrayal and subsequent mistrust. If it remains at the level of a tool or a feature (no anthropomorphosis), users perceive failures as only “unreliable,” and, in combination with good, transparent AI explanations, disappointment and a sense of unreliability can be avoided entirely.
It might be fun to choose your agent’s name and turn it into a character (anthropomorphize it), but this should not be taken as an arbitrary marketing decision. Companies must consider these disadvantages because they can significantly impact user interaction, perception, and trust.
Advice on Designing UX for AI and Chat Assistant
Now that we’ve covered some of the do’s and don’ts of these assistants’ most common features let’s cover some advice on designing them. I’ve found that keeping the user at the center of the design process and testing the UX through methods like prototyping always leads to the best result.
Keep users at the center of UX design
For a new interface to be clear and meet user needs, designers must always start with the user and the use case — who is the target group, what are their needs, their abilities, and challenges. The same approach must be taken when designing tools and functionalities around AI.
The example of the ChatGPT interface lacks focus on users and their needs – it is unclear who they are and what they should do. So users end up experimenting what to type to get a good result (whatever that means).
For entertainment, “trivial” tasks, or ideation, experimenting may be useful and desired. However, for serious work, such as making art, medicine, and data management, AI must become a partner and a tool. The initiative should remain with the user to evaluate the results, choose from alternatives, and guide the AI in the right direction. The control and decision-making stay with the user, who is ultimately responsible for the outcomes.
In the data management space, imagine a data steward accepting a business term suggestion regarding a number of attributes. They need to understand why it was suggested, and what the actual data or their profile looks like to make a decision. They won’t accept it without a deeper investigation. Similarly, in the data quality space, data stewards need to review data quality rules suggested by AI. Not doing so may result in incorrect reporting or even loss of customer data. They are the ones ultimately responsible for managing the data, so double-checking their work is paramount.
As you can see in the examples below, when users’ needs, use cases, and tasks become apparent, the AI becomes enveloped with a graphical UI to provide users with more control and explanation (or combines both approaches: chat + graphical interface).
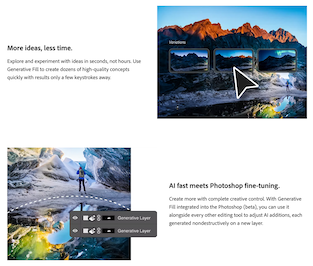
Adobe Photoshop suggests fill and area variations, leaving control over the final result to the artist.
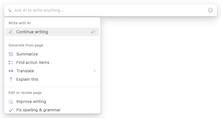
Notion suggests further actions in a text document, moving away from remembering the correct input/experimenting with the interface, to a traditional user interface designed around typical user actions.
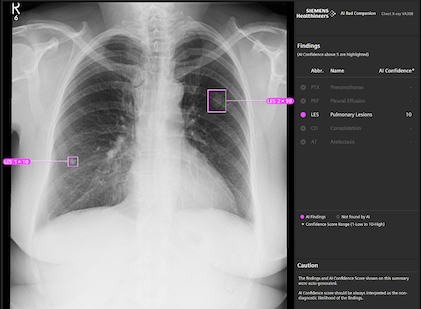
Siemens AI-Rad communicates confidence in findings to radiologists, using a traditional GUI as the user interface.
How to design UX for generative AI
The design process for these systems remains the same as before: user research, ideation, prototyping, and testing. These methods have been valid for decades, providing valuable and fast results.
It is easy to get carried away with AI and start implementing LLMs into all systems without thinking. Still, just like with all technologies that have come before, it is people who use them for their work and entertainment, and they should be at the center of product design and development.
Even prototyping, low-fi and hi-fi, has its place in designing for AI. Outputs from AI can be mocked up (in collaboration with engineers), and an application walkthrough can be prepared in prototyping tools as well as in HTML. We save time with the development of the final product, and, most importantly, with every subsequent test, we learn something new about the target group and how they will interact with the tools we create.
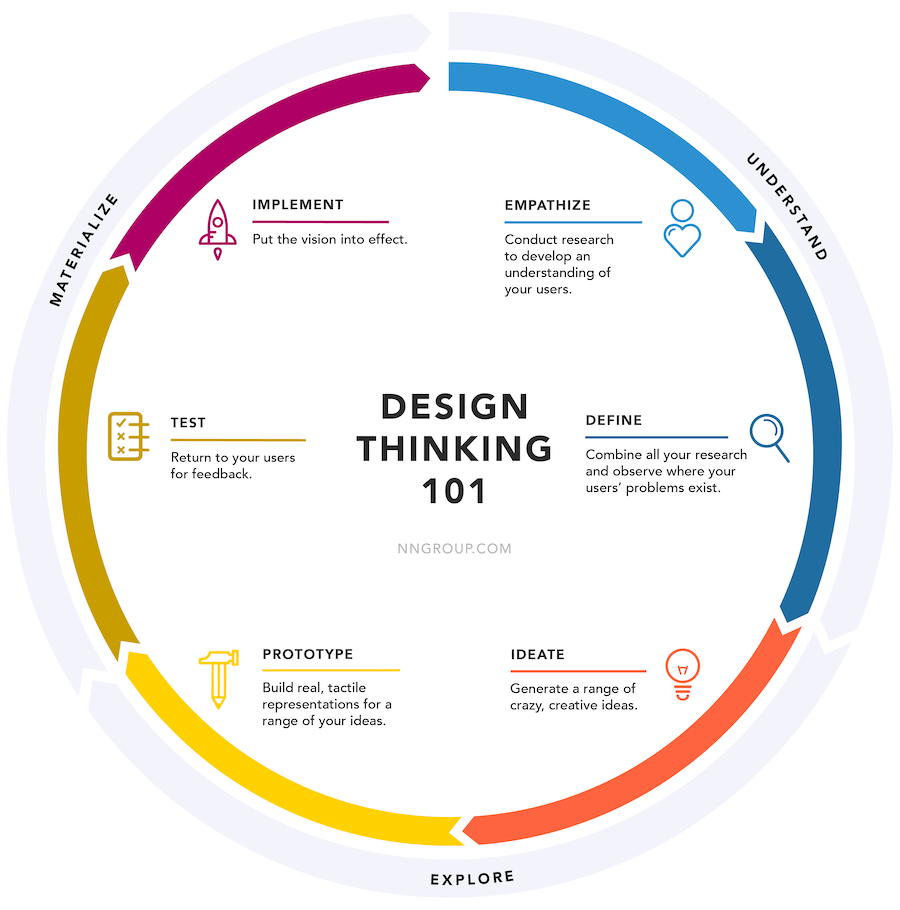
Good UX isn’t just important for AI. UX design for data management software (such as Ataccama) brings many benefits to our customers. Data stewards, analysts, and engineers do not have to struggle with complicated software – even business users, such as data owners, can find their way around. They are faster and more productive, spend less time training, and make fewer mistakes.
Good UX processes are an internal investment that always pays off for the product company. It develops features that make sense and provide value to users, customers, and development teams program-tested prototypes – and there is no need to fix as many usability problems in the code and pay off UX debt.
Takeaways
So, if you’re designing UX for an AI interface, start like with any other product. Start with scenarios (or JTBD, HMW, etc.), ideate, involve users in the design process, and test prototypes.
- For technical users and rigorous use cases: give maximum control to users, support user performance and decision-making, move AI into the position of an advisor, and explain the model, bias, and confidence of results transparently (e.g., human-in-the-loop approach).
- For non-technical and fun use cases: make it magical, a great result with one click, and give users the power to do tasks they would otherwise not be able to do.
- A cool name and chat interface are not always the best: If you have a clear use case, a classic “non-anthropomorphic” GUI is better and avoids all the challenges of a conversational interface (see above). However, Chat interfaces are suitable for arbitrary use cases (e.g., customer support, smart homes), and anthropomorphism improves user-friendliness.
If you’d like to learn more about Gen AI and its implications, you can visit our AI thought leadership page. Also, check out our fireside chat where industry experts (from OpenAI and T-Mobile) discuss this technology’s impact on data management.
Anja Duricic
Anja is our Product Marketing Manager of Data Quality and Data Governance at Ataccama. She is passionate about the human experience, learning about real-life companies, and helping them with their real-life needs.