Use cases for AI in the financial services industry
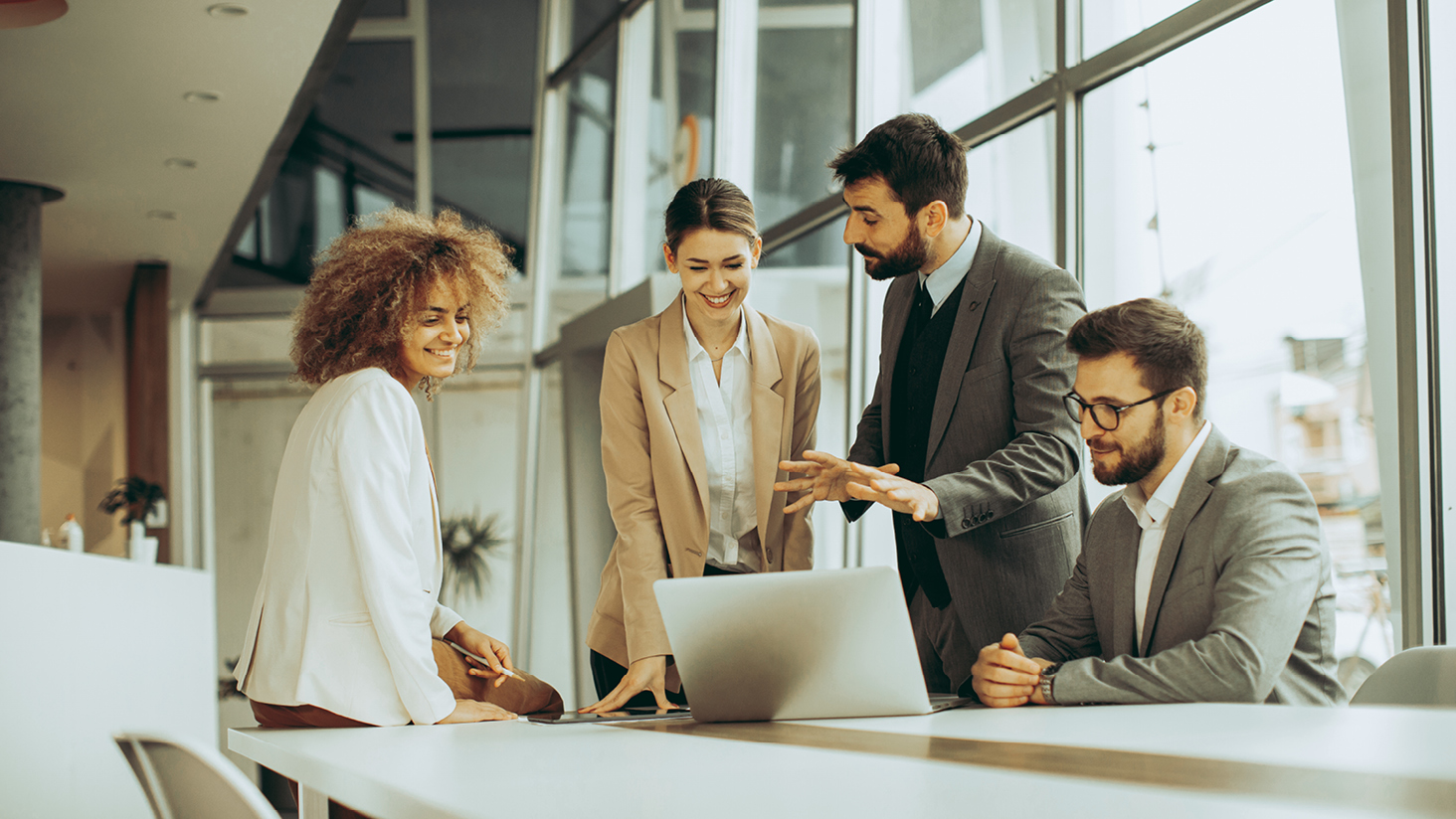
From credit underwriting to chatbots, AI’s capabilities are replacing traditional financial sector practices. However, a Citi survey revealed that 68% of respondent companies are still only in the ‘beginning’ stages of their AI journey (Citi).
Financial sector businesses at the forefront of AI are forming dedicated teams, with many GenAI leaders reporting directly to C-suite executives. This whitepaper aims to help you envision AI’s transformative potential and pinpoint strategic implementation opportunities.
By reading this whitepaper, you will gain insights to present AI strategies in executive meetings and demonstrate how six key AI use cases can advance your institution’s operations and competitiveness.
Anti-Money laundering / fraud
Traditional fraud detection systems often rely on static rule-based approaches, which struggle to adapt to new and evolving fraud tactics. According to an Association of Certified Fraud Examiners report, banks lose up to 5% of their revenue annually to fraudulent activities.
These systems can generate false positives, leading to inefficient resource allocation and customer dissatisfaction. Additionally, manual reviews of flagged transactions are time-consuming and prone to human error. The increasing sophistication of fraudsters necessitates more adaptive and responsive systems.
How can AI mitigate fraud?
AI-powered fraud detection uses machine learning algorithms to analyze transaction patterns and identify anomalies in real time. By leveraging historical data, AI models can predict potential fraud more accurately than traditional systems.
These systems continuously learn and adapt to new fraud patterns, ensuring they remain effective against emerging threats. AI also automates routine checks, allowing human analysts to focus on complex cases. Natural language processing can analyze unstructured data, such as emails and chat logs, for additional fraud indicators. By providing real-time alerts, AI systems help banks respond more swiftly to fraudulent activities. AI’s scalability allows it to handle large volumes of transactions without compromising accuracy.
What are the challenges to AI fraud detection?
Poor data quality can lead to ineffective models that fail to detect fraud accurately in the finance sector. Other challenges with AI fraud detection include:
- Balance in AI fraud detection models: An overly sensitive model can trigger a surge in costly investigations, while an insensitive one might fail to catch fraudulent claims, leading to significant financial losses.
- Legacy systems may not readily accommodate AI solutions: Requiring substantial IT resources and meticulous planning to ensure smooth operation.
- EU AI Act and other regulations: AI-driven fraud detection systems must adhere to these regulations, maintaining transparency and providing clear explanations for their decisions to build trust and maintain regulatory compliance.
AI fraud detection in finance: implementation, impact, and key players.
To understand the power of AI fraud detection, we turn to some of the world’s most highly credited institutions: Absa, one of the largest banks in Africa, and Mastercard.
- Absa: Partnered with SymphonyAI and reduced false positive alerts by 77% while capturing all suspicious activity found using their current transaction monitoring system. Absa identified 21 new risks compared to the slower manual adjustment of rules or reporting methods.
- Mastercard: Introducing an enhanced GenAI tool, Decision Intelligence Pro, for banks and financial institutions. Launching later this year, it aims to reduce transaction screening costs by up to 20%. The tool can analyze up to 1 trillion data points and deliver a safety score in under 50 milliseconds, potentially decreasing false positives by 85% (CNBC).
Credit risk & underwriting
As risks become increasingly diverse and complex due to factors like cyber threats, climate change, and other emerging scenarios, AI tools can enhance the ability of financial institutions to evaluate risk and underwrite policies with greater speed and accuracy.
AI-driven risk and underwriting systems can analyze extensive data from public sources, third-party transactions, historical records, and other channels, streamlining manual processes and reducing errors.
How can AI enhance credit risk and underwriting?
Traditional credit scoring relies heavily on structured financial data such as credit history and payment behavior, providing valuable but limited insights into a borrower’s creditworthiness. AI and natural language processing (NLP) can enhance this by analyzing unstructured data like rent payments, gig economy income, and social media activity. This broader data analysis improves approval rates and risk evaluation by offering a more comprehensive view of an individual’s or business’s financial situation.
Additionally, AI tools excel in identifying patterns and relationships within data that traditional models might miss, leading to more accurate credit risk assessments and reducing the likelihood of defaults and fraud. Advanced algorithms can also simulate scenarios based on historical data to predict credit risk more precisely, enhancing credit scoring and decision-making processes. Continuous monitoring with AI can further support this by tracking borrower behavior and macroeconomic conditions, aiding in loan loss provisioning and fraud detection.
AI-driven models also allow for customized credit risk assessments tailored to specific industries, customer segments, or regions, improving upon traditional broad-based risk modeling. This customization leads to more dynamic credit decisions and pricing. Additionally, AI can enhance lead management by analyzing diverse data sources to identify promising leads and create personalized products and services. Automation of routine processes through AI and robotic process automation also reduces documentation burdens, accelerates decision-making, and improves cost efficiency.
What are the challenges to using AI for credit risk and underwriting?
AI models demand vast quantities of accurate, clean, and seamlessly integrated data for reliable predictions. Discrepancies or gaps in this data can lead to flawed outcomes, potentially resulting in financial losses.
Learn more about the value of DQ for AI in our solution demo.
Other challenges facing AI in financial underwriting include:
- Interpretability: The complexity of AI algorithms can create transparency issues, making it difficult for underwriters to trust and understand AI-generated decisions, which is crucial in the financial sector’s regulatory landscape.
- Explainability: Decision-making processes need to be explainable to meet regulatory and compliance requirements in the financial industry, ensuring transparency and accountability.
- False Positives/Negatives: The risk of AI models producing false positives or negatives in risk assessment could lead to suboptimal underwriting decisions, highlighting the need for a human-in-the-loop system in financial decision-making.
AI for credit risk and underwriting: implementation, impact, and key players
One AI company leading the way for AI-improved credit risk and underwriting is Zest AI.
- Zest AI: Enabled Commonwealth Credit Union to approve more members and reduce charge-off and delinquency rates. With 70-83% of consumer loan decisions automated, Commonwealth’s competitive edge has significantly improved. Since 2021, Zest AI has facilitated the approval of over $324 million in consumer loans, totaling more than 18,000 loans. The performance of these loans is notably stronger, with delinquency rates 30-40% lower than those underwritten using traditional scoring methods (Zest AI).
Personalized member services/customer targeting
Financial institutions traditionally target customers using demographic and geographic analysis, transactional data, and customer surveys. They rely on direct mail, telemarketing, and community events to reach potential clients. Cross-selling to existing customers and partnerships with local businesses are also common strategies. While these methods have been effective, innovators see them as outdated and less efficient than AI-driven targeting.
How can AI help banks target customers?
AI can help credit unions offer personalized services to their members. By analyzing data on member behavior and preferences, AI systems can provide tailored financial advice, recommend relevant products, and customize communication, enhancing member satisfaction and loyalty. For example, AI-driven chatbots can provide instant support and personalized financial guidance based on a member’s transaction history and financial goals.
What are the challenges to implementing AI for customer targeting?
AI algorithms depend on high-quality, unbiased data for accurate customer segmentation and targeting; poor data quality or algorithmic bias can lead to flawed insights and ineffective marketing strategies.
Beyond that, AI-driven targeting in banks and credit unions faces several unique challenges.
- Integrating AI tools with existing CRM and marketing automation: These platforms can be complex and costly, requiring seamless scalability and real-time data synchronization.
- Ensuring compliance: Regulations like GDPR and CCPA are crucial, as companies must implement robust data governance practices to protect Personally Identifiable Information (PII) and avoid breaches.
- Losing humanity: Over-reliance on AI also risks diminishing the personal touch in customer interactions, potentially reducing brand loyalty and engagement.
AI for finance customer targeting: implementation, impact, and key players
Ping An Bank, a Chinese joint-stock commercial bank with its headquarters in Shenzhen, implemented an AI-driven prediction algorithm, demonstrating a clear ROI in customer targeting.
- Ping An Bank: By analyzing each customer’s needs and predicting the optimal product-per-customer (PPC) ratio, the algorithm identifies gaps where customers are underutilizing products. For example, if a customer’s ideal ratio is eight products but they only use two, the system prompts the relationship manager to cross-sell or upsell relevant products proactively.
This AI-powered approach enables banks to create highly personalized service experiences by segmenting customers into microsegments, delivering timely, tailored offers, and enhancing interactions across various channels. As a result, relationship managers receive actionable insights that boost productivity, while customers benefit from streamlined, intuitive service and relevant product recommendations (AsiaMoney).
Customer service chatbots
Customer satisfaction and timely responses are paramount in the financial sector. Manually handling inquiries often strains resources and can result in uneven service quality. Large Language Model (LLM) chatbots offer a solution by streamlining customer interactions, ensuring consistent, prompt, and accurate responses across the entire organization.
How can using an AI chatbot improve customer service?
AI chatbots offer continuous support, enhancing customer satisfaction by operating round-the-clock. They handle various queries independently, allowing human agents to focus on more complex issues.
Chatbots significantly reduce response times, providing instant assistance for routine tasks like balance inquiries and transaction history. They collect customer information at the start, ensuring quick and efficient interactions.
Additionally, AI chatbots use customer data and preferences to deliver tailored solutions and recommendations. They ensure a consistent experience across channels and provide accurate and relevant information on any platform.
What are the challenges to implementing a chatbot for customer service?
These chatbots rely on accurate data to understand customer queries, provide relevant information, and offer personalized solutions. Without high-quality data, chatbots risk providing incorrect or misleading information, eroding customer trust and potentially leading to costly mistakes. Investing in data quality ensures that chatbots deliver better results.
Beyond data quality, some other challenges for customer service chatbots include:
- Complex queries: Chatbots often struggle to understand complex or ambiguous queries, leading to user frustration and decreased customer satisfaction.
- Ensuring discoverability and creating engaging experiences: Anticipating user needs and predicting behavior is crucial, such as recognizing when a customer inquires about credit card payments. Chatbots must provide personalized, meaningful interactions beyond generic responses, focusing on financial literacy and connectivity to banking systems.
- Hallucinations: AI models are known to hallucinate (generating information that does not exist) which can lead to significant financial and reputational risks if chatbots operate autonomously. Therefore, before deploying AI in customer-facing roles, banks should invest in robust AI usage policies, frameworks, and architecture.
Human oversight is indispensable to monitor interactions and maintain compliance with legal and business standards. In fact, an increased human presence may be necessary to ensure AI chatbots operate within these guardrails.
AI customer service chatbots for finance: implementation, impact, and key players
Several financial institutions have already reaped the benefits of chatbot implementation, demonstrating the technology’s effectiveness in improving efficiency and customer satisfaction including Meriwest Credit Union and Bank of America:
- Meriwest Credit Union: Implemented Kasisto, which resulted in a 30% increase in the profitability of their client base that engages with the AI chatbot (Kasisto).
- Bank of America: More than 98% of clients get the answers they need from Erica, their custom AI chatbot, within 44 seconds, on average (BofA).
Improving customer experience
Banks and credit unions interact with tens of thousands of clients every month. Even with a large team of human assessors, they can only monitor and review less than 1% of these interactions. Existing monitoring and oversight processes are slow, time-intensive, and random, with the monitoring team listening to whole, often lengthy, client calls.
How can using an AI improve customer experience?
AI and NLP can revolutionize the monitoring and review of client interactions at financial institutions. They allow for a significantly higher percentage of interactions to be analyzed compared to the less than 1% typically reviewed by human assessors. These technologies can automatically transcribe and analyze calls, emails, and chat conversations, identifying key themes, sentiments, and specific issues in real time.
This enables negative experiences to be quickly addressed, ensuring compliance, and providing more consistent and objective quality assurance. Sentiment analysis helps understand customer emotions, leading to tailored responses and improved customer satisfaction. Models that analyze voice and speech characteristics can match agents with customers based on behavioral and psychological mapping. Similarly, transcript analysis can enable the prediction of customer distress and suggest resolutions to the agent.
What are the challenges to implementing AI to improve customer experience?
- Substantial investment in integration efforts: Banks and credit unions often have legacy systems that may not be compatible with new AI technologies.
- Sensitive customer information: Compliance with regulations such as GDPR and other data protection laws necessitates robust security measures, which can be complex and costly.
- Accuracy and reliability of AI and NLP algorithms: These systems rely on large amounts of high-quality data to function effectively, and any biases or inaccuracies in the data can lead to erroneous insights and decisions.
AI customer experience: implementation, impact, key players
Cavendish Online, part of Lloyds Banking Group, used AI to enhance their customer experience:
- Cavendish Online: Teamed up with Aveni.ai to become a pioneer in utilizing AI technology for customer protection services. Aveni’s platform, using Generative AI and Natural Language Processing (NLP), analyzes customer calls to improve service quality and identify vulnerabilities. While advisers will still lead calls, the Aveni system can process a day’s worth of calls in minutes, providing insights to enhance service and target skill development for advisers. This collaboration aims to significantly improve the customer experience by leveraging AI for real-time feedback and continuous improvement (Lloyd’s Banking Group).
Data quality as a foundation for AI
Nearly all AI use cases in the financial industry share a common challenge: the quality of data currently available within the business. Accurate assessments and effective utilization of AI depend heavily on high-quality data.
However, many financial institutions struggle with data that is incomplete, inconsistent, or outdated. To fully harness the potential of AI, decision-makers must prioritize improving data quality first and foremost. This involves:
- Implementing robust data governance practices
- Investing in advanced data integration and cleaning technologies
- Fostering a culture that values data accuracy and completeness.
Only by addressing these foundational issues can financial institutions truly begin to use AI to enhance their member risk assessments, streamline loan processing, and optimize financial product offerings. We at Ataccama are leaders in helping banks and credit unions use high-quality data in a secure and governed way. Download this free ebook to begin building your end-to-end DQ framework.