AI use cases for the insurance industry
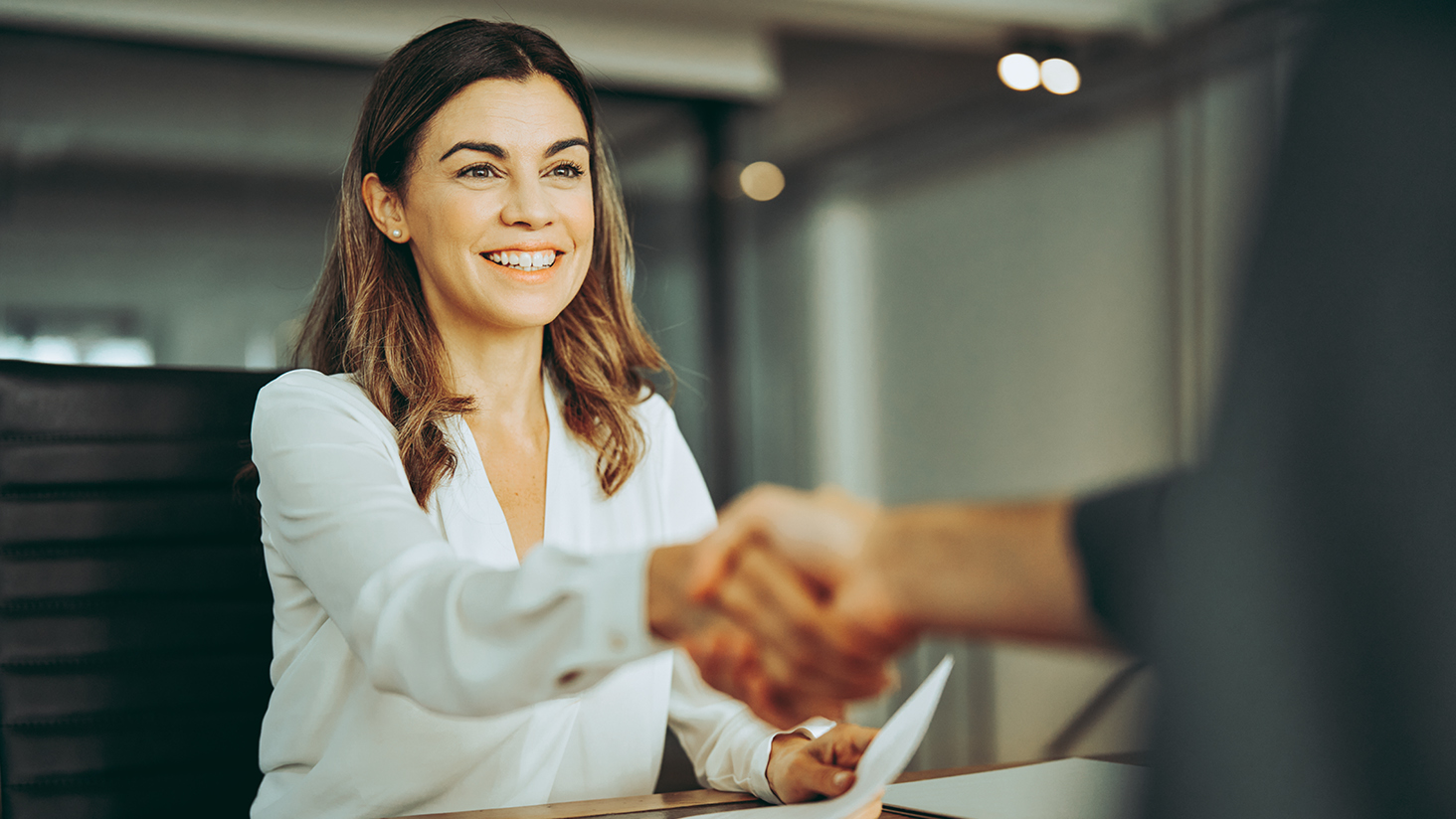
From underwriting and claims processing to fraud detection and personalized policy offerings, AI’s capabilities are gaining momentum to replace traditional insurance practices. 42% of insurers are already investing in GenAI, and 57% plan to (EY).
Insurers are building AI-dedicated teams, with over a quarter of GenAI leaders reporting directly to C-suite executives. We want to help you envision AI’s transformative potential and identify opportunities for strategic implementation.
After reading this whitepaper, you will be equipped with the knowledge to present AI strategies in the boardroom and demonstrate how six AI use cases can propel your business forward.
Underwriting & risk assessment
Traditional risk assessment methods are often inefficient and rely on limited data, leading to inaccurate insurance policy pricing and increased risk for insurers. AI can speed up the process and save insurers time and budget.
How can AI improve underwriting processes?
AI can analyze diverse datasets (e.g., health records, financial data, and historical claims data) to more accurately assess risk and underwrite policies. Machine learning models can also more effectively predict the likelihood of claims than traditional methods. For health insurance companies, AI can analyze medical records, lifestyle data, and historical claims.
An excellent example of these benefits is Daido Life Insurance in Japan. They have developed an impressive AI-driven underwriting model that visualizes the decision-making process, allowing underwriters to assess cases while reviewing the AI’s predictions and cautionary points. This approach not only enhances back-office efficiency but also addresses AI’s “black box” issue by enabling human verification of AI results.
What are the challenges to AI underwriting?
A major challenge to using AI for underwriting is ensuring the quality and consistency of data; AI models require vast amounts of accurate, clean, and well-integrated data to make reliable predictions, yet discrepancies or gaps in data can lead to flawed outcomes.
According to a survey conducted by EY, 57% of insurance executives cite data quality and availability as the biggest challenge in implementing AI and machine learning solutions. (EY)
Learn more about the value of DQ for AI in our solution demo.
Other challenges for AI in underwriting include:
- The complexity of AI algorithms can make them difficult to interpret, creating transparency issues and making it hard for underwriters to trust and understand AI-generated decisions.
- Protecting against AI bias is another significant challenge. It can lead to unfair or discriminatory underwriting decisions. AI systems must adhere to stringent data privacy and security standards. Decision-making processes need to be explainable to meet regulatory and compliance requirements.
- The risk of AI models producing false positives or negatives in risk assessment could lead to suboptimal underwriting decisions, making the case for a human-in-the-loop system.
AI underwriting & assessment: implementation, impact, and key players
To see the real ROI of AI for underwriting, let’s examine a few case studies.
- Markel (working with underwriting AI vendor Cytora): Experienced an uplift of 113% in productivity in their underwriting teams and a reduction in SLA quote turnaround time for strategic partners from 24hrs to 2hrs. (Cytora)
- Haven Life (a digital-first, direct-to-consumer life insurance company): Implemented AI-driven underwriting systems that can issue policies in as little as 20 minutes, compared to the weeks or months typically required for traditional underwriting.
- MassMutual (Massachusetts Mutual Life Insurance Company): Since implementing predictive modeling, the time to policy issuance has decreased by 20% for applicants in the healthiest risk class. The number of applicants declining to purchase policies also reduced by 25% when the decision was made by a predictive model compared with traditional underwriting.
Claims processing automation
Manual claims processing is inherently time-consuming and error-prone, significantly impacting operational efficiency and customer satisfaction. On average, processing a single insurance claim can take several weeks, leading to frustration among policyholders and increasing the likelihood of customer churn.
The labor-intensive nature of manual processing results in higher operational costs, while human errors can lead to delayed settlements, further exacerbating customer dissatisfaction.
How can AI improve claim processing?
AI has the potential to revolutionize claims processing by automating the entire workflow from initial submission to final settlement. Specifically, companies can use Natural Language Processing (NLP) to read and interpret claim documents, extracting relevant information quickly and accurately.
Machine learning algorithms can then assess the validity of claims by comparing them against historical data and identifying patterns indicative of fraud or inaccuracies. By automating these steps, AI can significantly reduce the time required to process claims, often from weeks to hours.
Moreover, AI can ensure consistency and accuracy in document handling, minimizing the errors commonly associated with manual processing. Insurers adopting AI-driven claims processing have reported reductions in processing times and decreased operational costs. Increased efficiency and accuracy also enhance customer satisfaction and improve retention rates.
What are the challenges to AI claim processing?
Ensuring accuracy in document interpretation and processing is critical to avoid errors. High-quality, diverse datasets are always needed to train AI models effectively. Beyond that, there’s also data privacy and security maintenance, particularly with the sensitive information involved in insurance claims. Integrating AI solutions with existing applications can also be complex and resource-intensive
Addressing these challenges requires a strategic approach involving robust data governance, comprehensive employee training for new technologies, and a clear integration plan to align AI initiatives with existing business processes.
AI claims processing: implementation, impact, and key players
For this benefit, you can count on high expected ROI: Major cost savings in operational expenses, improved customer satisfaction, and faster claim settlements leading to higher retention rates.
Some of the more prominent vendors in this space are:
- Sprout.ai: Automating claim processing for major insurers like Zurich UK, which saw a 98% accuracy rate in the 2,000 claims processed in the pilot (Sprout).
- Simplifai: Claims to reduce operational expenditure on manual processing by 50% (Simplifai).
Detecting fraudulent claims
Insurance fraud is a pervasive issue, causing substantial financial losses for the insurance industry globally. In the United States alone, fraudulent activities account for an estimated 10% of the property-casualty insurance industry’s incurred losses and loss adjustment expenses (NICB).
This equates to over $30 billion annually, a significant burden on the industry that translates into higher consumer premiums and reduced profitability for insurance companies (Insurance-Canada).
As you may know, fraudulent claims with staged losses or exaggerated damages contribute heavily to these profit losses. As fraud schemes become increasingly sophisticated, more effective detection and prevention methods are critical.
How can AI mitigate fraud?
AI offers a powerful solution to the problem of insurance fraud by enabling the analysis of vast amounts of data to detect patterns and anomalies that indicate fraudulent activity. Machine learning algorithms can identify suspicious claims by comparing them against known fraud patterns and detecting subtle anomalies that might escape human detection.
These models allow insurers to flag suspicious claims for further investigation quickly and accurately. Furthermore, AI can continuously learn and adapt to new fraud tactics, making it a robust tool in the ongoing fight against fraud.
AI can also streamline the claims process, reducing the time required to process legitimate claims and improving customer satisfaction. By integrating AI into their systems, insurers can achieve a more proactive and preventive approach to fraud detection.
What are the challenges to AI fraud detection?
Poor data quality can lead to ineffective models that fail to detect fraud accurately. The Coalition Against Insurance Fraud surveyed 30 insurers, and 52% indicated data quality as their primary concern when adopting AI in their operations (CAIF).
Other challenges with AI fraud detection include:
- Balancing the model’s sensitivity: An overly sensitive model can increase operational costs due to unnecessary investigations, while an insensitive model can allow fraudulent claims to slip through.
- Adapting to existing infrastructure: Integrating AI systems with existing claims processing infrastructure can also be complex and require significant IT resources and planning.
- Regulatory compliance and data privacy: Concerns must be addressed, particularly with the advent of comprehensive AI regulations like the EU AI Act. AI systems must be transparent and their decisions explainable.
AI fraud detection: implementation, impact, and key players
Insurers experience a significant reduction in fraudulent claims, resulting in cost savings and improved accuracy. AI can minimize unnecessary investigations and enhance operational efficiency.
Some valuable vendors to keep in mind:
- Shift AI: Have partnered with MetLife Japan, claiming to find 3x more fraud than manual or rules-based tools (MetLife).
- FRISS: Uses AI to analyze claims data for patterns indicative of fraudulent activity, helping insurers reduce false positives and improve investigation efficiency (FRISS).
Dynamic pricing models
Traditional static pricing models can lead to mispriced policies, either too expensive (losing customers) or too cheap (increasing risk exposure). AI can lead to more dynamic pricing that can shift to fit business needs.
How can AI facilitate dynamic pricing for insurance policies?
AI can develop dynamic pricing models that adjust in real time based on various factors, such as customer behavior, market trends, and risk assessments, ensuring competitive yet profitable pricing.
While instant quotes have long been a feature in insurance, the maturation of pricing algorithms and the proliferation of connected devices like telematics and IoT sensors have dramatically increased the amount of data available. This data explosion allows insurers to tailor policies more precisely to individual customers.
Traditional rules-based systems are limited in their ability to adjust for unforeseen events. In contrast, advanced AI platforms can leverage decision-making tools, such as “what-if” scenarios, to simulate data-driven outcomes and predict pricing strategies with the highest success rates.
These sophisticated platforms can calculate millions of rates and product options daily by analyzing day-to-day behaviors, allowing businesses to scale effortlessly while offering greater personalization and convenience to consumers.
What are the challenges to dynamic pricing?
Dynamic pricing relies heavily on large volumes of high-quality data. Only accurate, complete, and updated data can lead to correct pricing decisions. Insurers need robust data governance practices and advanced data integration systems to ensure the accuracy and completeness of their data sources.
Companies can also face challenges with dynamic pricing for their customers. Customers may perceive dynamic pricing as unfair or discriminatory, especially if they do not understand the factors influencing their premiums.
Clear communication and education about dynamic pricing and its benefits to customers can help mitigate these concerns. Providing tools for customers to see how their behavior influences pricing can also build trust through transparency.
AI dynamic pricing: implementation, impact, and key players
Although the initial investment in dynamic pricing systems can be substantial (whether through outside vendors or deploying an in-house solution), these solutions often prove more cost-effective than static pricing in the long run.
Single, end-to-end insurance platforms integrating iterative deployment with rate-making and execution capabilities provide insurers with a significant competitive edge, even in challenging market conditions.
The ability to respond in real time to consumer preferences and behavioral changes while maintaining compliance and governance over the pricing process allows insurers to realize value almost immediately.
One vendor to keep in mind:
- Akur8 specializes in AI-driven insurance pricing automation: Their platform allows insurers to build transparent and auditable pricing models, improving accuracy and speed in pricing decisions. Another vendor called Earnix claims that their dynamic pricing model led to a 15% increase in quote conversions at a large US auto insurer (Earnix).
Customer service chatbots
Handling customer queries manually requires significant human resources and can lead to inconsistent service quality and slower response times. LLM chatbots can expedite this process, leading to more consistent customer service organization-wide.
How can using an AI chatbot improve customer service?
AI-powered chatbots can handle a large volume of customer inquiries 24/7, providing instant responses and improving customer satisfaction. They can answer common questions, guide customers through processes, and even assist in filing claims.
Don’t believe the lousy reputation people give “talking to a robot.” 74% of consumers are willing to use robo-advice to purchase insurance, highlighting the demand for AI-driven, customer-centric underwriting solutions (Accenture).
“Members reach out to us often at the most vulnerable moments in their lives. This collaboration shows that providing AI-driven and timely interventions for members can enhance care coordination, enrich their experience, and decrease complaints,”
Somesh Nigam, Ph.D., Blue Cross senior vice president and chief analytics and data officer
What are the challenges to implementing a chatbot for customer service?
While chatbots have many benefits, they’re not the easiest models to implement effectively. Some of the challenges include:
- Regular updates: Companies must continuously update the chatbot’s knowledge base.
- Human-agent transitions: When necessary, seamless transitions to human agents are pivotal for customer satisfaction.
- Need for human connection: Despite their efficiencies, customers are still significantly dissatisfied with “speaking to a robot.”
AI chatbots for insurance: implementation, impact, and key players
This is a relatively low investment cost with potentially high returns from savings on operational costs with manual representatives. Some interesting case studies of successful chatbot implementations:
- MetLife: Implemented Cogito AI to analyze conversations in real time, which improved first-call resolutions by 3.5%, and increased customer satisfaction score by 13%, all while cutting the average call time in half (Time).
- Clearcover (an auto insurer): experimented with AI agents to answer queries. Over 35% of Clearcover’s customer chat inquiries were automatically resolved within the first month of launching Ada’s AI Agent for customer service (LIN).
Catastrophe modeling and risk management
According to a Swiss Re report, 285 natural catastrophe events occurred in 2022, causing the global economy $284 billion, well above the 10-year average of $220 billion. Natural disasters and catastrophic events can result in significant financial losses for insurers.
Traditional models may not provide accurate predictions, leading to inadequate risk management. However, new technology is becoming increasingly accurate at predicting the occurrence and outcomes of these events.
How can AI improve catastrophe modeling?
AI can enhance catastrophe modeling by analyzing diverse datasets, including weather patterns, historical disaster data, and geographical information, to more accurately predict the impact of future events.
What are the challenges to modeling catastrophes using AI?
Catastrophes are, by nature, rare and unpredictable events. Modeling such events accurately is inherently challenging due to the lack of frequent occurrence data and the high degree of uncertainty involved. Probabilistic models, stress testing, and scenario analysis can help insurers better prepare for impactful events.
Accurate catastrophe modeling requires extensive historical data, including weather patterns, geographical data, and previous loss data. This data is often incomplete, inconsistent, or unavailable in the necessary granularity.
AI catastrophe modeling: implementation, impact, and key players
Returns will vary based on investment scale, model accuracy, and integration effectiveness. Benefits include improved risk assessment, leading to better pricing strategies and reduced losses through more effective risk mitigation.
Additionally, better models enable optimized capital allocation, ensuring reserves are appropriately matched to risk exposures. They also help meet regulatory requirements, preventing costly fines.
Some interesting vendors for catastrophe modeling:
- Zesty.ai: Assess property risk from natural disasters such as wildfires, hurricanes, and floods. They use high-resolution imagery and machine learning to provide detailed risk assessments.
- Kensho: Analyze and predict the impact of various catastrophe scenarios. Their models are used for financial risk assessment, including insurance and reinsurance markets.
- HazardHub (by GuideWire): Provides detailed hazard risk data, offering insights into risks from floods, wildfires, earthquakes, and other natural hazards, helping insurers to better understand and price their policies.
Data Quality as a foundation for AI
Nearly all AI use cases in the insurance industry share a common challenge: the quality of data currently available within the business. Accurate assessments and effective utilization of AI depend heavily on high-quality data.
However, many insurance companies struggle with data that is incomplete, inconsistent, or outdated. To fully harness the potential of AI, decision-makers must prioritize improving data quality first and foremost. This involves:
- Implementing robust data governance practices
- Investing in advanced data integration and cleaning technologies
- Fostering a culture that values data accuracy and completeness.
Only by addressing these foundational issues can insurers truly begin to use AI to enhance their risk assessments, streamline claims processing, and optimize pricing strategies. We at Ataccama are leaders in helping insurance companies use high-quality data in a secure and governed way. Download this free ebook to begin building your end-to-end DQ framework.